Senior Editor
- FMA
- The Fabricator
- FABTECH
- Canadian Metalworking
Categories
- Additive Manufacturing
- Aluminum Welding
- Arc Welding
- Assembly and Joining
- Automation and Robotics
- Bending and Forming
- Consumables
- Cutting and Weld Prep
- Electric Vehicles
- En Español
- Finishing
- Hydroforming
- Laser Cutting
- Laser Welding
- Machining
- Manufacturing Software
- Materials Handling
- Metals/Materials
- Oxyfuel Cutting
- Plasma Cutting
- Power Tools
- Punching and Other Holemaking
- Roll Forming
- Safety
- Sawing
- Shearing
- Shop Management
- Testing and Measuring
- Tube and Pipe Fabrication
- Tube and Pipe Production
- Waterjet Cutting
Industry Directory
Webcasts
Podcasts
FAB 40
Advertise
Subscribe
Account Login
Search
How digital twins add a new level of intelligence in metal fabrication
Perfecting how people and technology interact in manufacturing settings
- By Tim Heston
- August 17, 2023
- Article
- Shop Management
Imagine a plant manager arriving in the morning, opening a laptop or a phone app, and asking, “What should I pay attention to today?” The system then would present potential problems. A machine is down unexpectedly. A job from the previous shift took longer than expected. Perhaps a department is short a few people. The system suggests changes, and the plant manager accepts or adjusts them as needed.
Such a scenario isn’t science fiction. In fact, the technology could become real as soon as next year. At this writing, Ultisim, a Chapel Hill, N.C.-based firm specializing in digital twins, is working with a manufacturer to develop such a system, tying together data from machines, enterprise resource planning platforms, and a plethora of other sources. It uses a large-language-model interface similar to the one made famous by ChatGPT, but focused on solving problems manufacturers face.
“Every order [this manufacturer] gets is custom,” said Richard Boyd, CEO of Ultisim and its sister company Tanjo, which focuses on the algorithmic building blocks of machine learning and artificial intelligence (AI). “All that variety is what makes it hard and interesting. If we solve their problems, we can address other problems much easier.”
Such high-product-mix models are in the realm of custom metal fabrication, which is one reason Boyd plans to bring his solution to that market (he’ll be speaking in September in Chicago at FABTECH). At most custom fab shops, every job can have a specific routing through specific value streams; machines; or process-centric departments like cutting, bending, hardware, welding, powder coating, and assembly; with (depending on the job routing) a variety of secondary operations sprinkled in between.
Everyone in the organization can view the digital twin through what Boyd called “lenses” tailored for specific people and job functions. Someone in finance would have a lens that’s different from someone in sales, scheduling, or production planning.
Moreover, the data that builds the twin wouldn’t be moved from its original location. “We don’t need one big data lake,” Boyd said, adding that the system can pull what it needs and “leave the data where it is.” The last thing a company wants is to copy data over to a central location, since the act of copying gives you multiple versions of the same data and leads to all sorts of unneeded complexity.
Making all this happen calls for several key ingredients, the first being digitized data. Yes, most fabricators have modern machines that capture plenty of data, but they also probably still rely on old, simple machines in a few departments. Old iron dies hard, and mechanically speaking, they still get the job done well. They will, however, require additional sensors to digitize what they do (how many strokes, feet, or pieces per minute or hour for this or that job), but this really isn’t a challenge, especially now.
“We’ve had a revolution in sensors,” Boyd said, adding that some types are now being printed—not by 3D printers, but by relatively ancient dot-matrix technology. Even optical sensors are inexpensive, so adding them to old machines isn’t much of an economic or even technical hurdle anymore.
What has been a hurdle, Boyd explained, is what he called “dark data.” That includes data sitting within isolated software, unshared and underutilized. Boyd has spent years working with companies across various industries, including those with defense contracts that demanded completely transparent application protocol interfaces (APIs). This transforms dark data into useful information. Information, Boyd said, is “data at rest” or “data in motion,” which has been meta-tagged digitally for AI and machine-learning consumption.
Plenty of organizations use a hodgepodge of different systems at different facilities acquired over the years. Manufacturers are known for this, of course, but so are plenty of other industries. “In hospitals, for instance, people buy all these unintegrated systems, which sometimes don’t even agree on what time it is, and they’re not sharing the data,” Boyd said.
He added that some systems providers today charge significant fees for the privilege of complete data access. That practice might wane, along with other technical hurdles, as the market understands the value of data. Boyd said he sees market demand shifting as the value and financial returns become evident. His organization is predicting returns that dwarf the initial investment, 10 times or greater in some cases.
To make this happen requires not just data from machinery and software systems, but also from people. This includes exactly how people perform their jobs—specifically, how they move around and interact with technology. Boyd called this the “human choreography.”
Imagine a shop simulation that tests a specific job sequence from cutting to bending to welding. The simulation could include secondary operations like flat-part deburring and perhaps even hardware insertion. On that simulation, planners could see what tools operators would need to change out, where those tools are stored, how quickly on average changeovers could be made, and how changing the sequence could affect overall flow. The digital twin could even predict the ripple effects in other areas of the plant, depending on particular job mixes, as well as the bottlenecks—which, in high-product-mix production, can change with every new mix of jobs on the floor.
Tracking and optimizing human movement might seem a tad “Big Brotherish,” Boyd said, but he added that when a company links improvements to the right incentives, the culture can benefit. For instance, Ultisim’s test case in manufacturing happens to have an employee stock ownership program (ESOP), so as the company’s finances improve, employees see the benefit.
They also see how valuable their knowledge really is, considering it’s being built right into the system. Boyd emphasized that using a digital twin doesn’t eliminate the need for tribal knowledge. In fact, tribal knowledge isn’t inherently a bad thing. After all, a competitor can buy a similar machine or software platform, but they can’t duplicate someone with decades of experience.
Here, AI and digital twins promote decision-making based on both hard data and tribal knowledge. In the current test case, a production planner who’s nearing retirement reviews the decisions the digital twin suggests, then tweaks them as necessary. Those tweaks are then fed back into the system, results are analyzed, and—with knowledge from people and data from systems and machines—improvements are made.
Capturing that tribal knowledge has become critical for training the new generation. Marry it with actionable information, and you give younger workers the tools they need to thrive. They’re not doing a job—bend this part, insert this hardware—because their supervisor told them to do it. Seeing the big picture, viewed through a specific lens and brought to life by the digital twin, they know that what they’re doing now is likely better than what came before. If it isn’t, they will learn, as will the digital twin, and the effort toward improvement continues. All in all, that’s not a bad way to spend a career.
About the Author
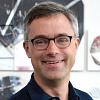
Tim Heston
2135 Point Blvd
Elgin, IL 60123
815-381-1314
Tim Heston, The Fabricator's senior editor, has covered the metal fabrication industry since 1998, starting his career at the American Welding Society's Welding Journal. Since then he has covered the full range of metal fabrication processes, from stamping, bending, and cutting to grinding and polishing. He joined The Fabricator's staff in October 2007.
subscribe now
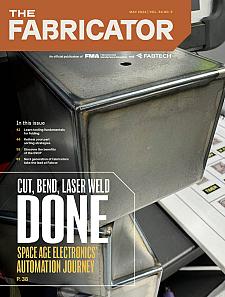
The Fabricator is North America's leading magazine for the metal forming and fabricating industry. The magazine delivers the news, technical articles, and case histories that enable fabricators to do their jobs more efficiently. The Fabricator has served the industry since 1970.
start your free subscription- Stay connected from anywhere
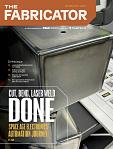
Easily access valuable industry resources now with full access to the digital edition of The Fabricator.
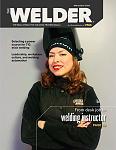
Easily access valuable industry resources now with full access to the digital edition of The Welder.
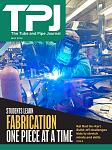
Easily access valuable industry resources now with full access to the digital edition of The Tube and Pipe Journal.
- Podcasting
- Podcast:
- The Fabricator Podcast
- Published:
- 04/16/2024
- Running Time:
- 63:29
In this episode of The Fabricator Podcast, Caleb Chamberlain, co-founder and CEO of OSH Cut, discusses his company’s...
- Trending Articles
Tips for creating sheet metal tubes with perforations
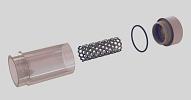
Are two heads better than one in fiber laser cutting?
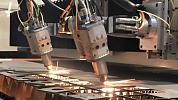
Supporting the metal fabricating industry through FMA
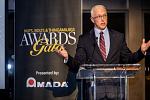
JM Steel triples capacity for solar energy projects at Pennsylvania facility
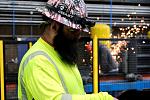
Omco Solar opens second Alabama manufacturing facility
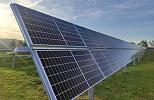
- Industry Events
16th Annual Safety Conference
- April 30 - May 1, 2024
- Elgin,
Pipe and Tube Conference
- May 21 - 22, 2024
- Omaha, NE
World-Class Roll Forming Workshop
- June 5 - 6, 2024
- Louisville, KY
Advanced Laser Application Workshop
- June 25 - 27, 2024
- Novi, MI