President
- FMA
- The Fabricator
- FABTECH
- Canadian Metalworking
Categories
- Additive Manufacturing
- Aluminum Welding
- Arc Welding
- Assembly and Joining
- Automation and Robotics
- Bending and Forming
- Consumables
- Cutting and Weld Prep
- Electric Vehicles
- En Español
- Finishing
- Hydroforming
- Laser Cutting
- Laser Welding
- Machining
- Manufacturing Software
- Materials Handling
- Metals/Materials
- Oxyfuel Cutting
- Plasma Cutting
- Power Tools
- Punching and Other Holemaking
- Roll Forming
- Safety
- Sawing
- Shearing
- Shop Management
- Testing and Measuring
- Tube and Pipe Fabrication
- Tube and Pipe Production
- Waterjet Cutting
Industry Directory
Webcasts
Podcasts
FAB 40
Advertise
Subscribe
Account Login
Search
Minding your P’s, Q’s, R’s--and revenue too
Part 3 in a series: A product mix, quantity, routing, and revenue analysis can jump-start a job shop’s improvement efforts
- By Shahrukh Irani, Ph.D.
- May 11, 2017
- Article
- Shop Management
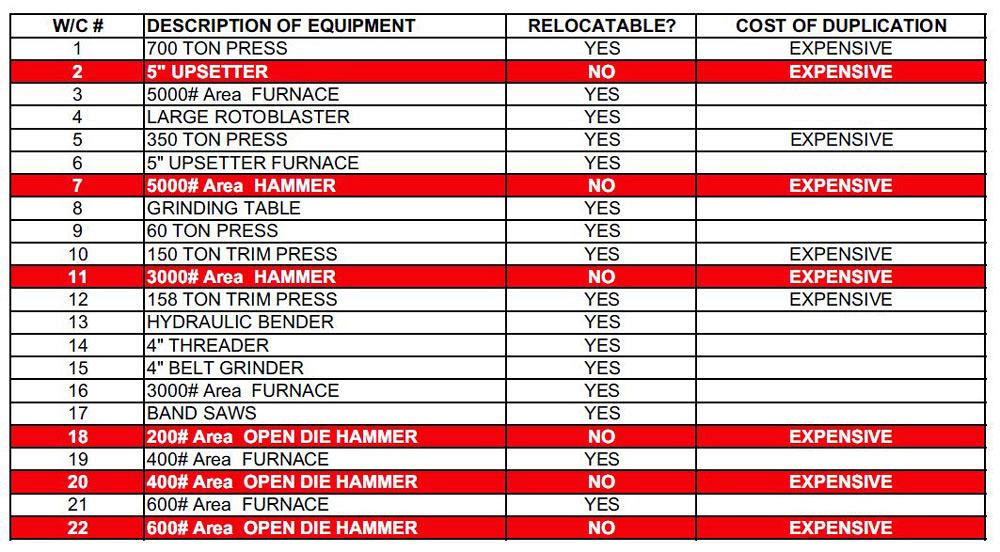
Figure 1
The company lists its work centers (W/Cs) and specifies whether the machine can be relocated and if the process would be expensive to duplicate. This is just a portion of the complete list of 57 work centers. The red signifies monuments, which aren’t practical to move. Highlighted green sections (not shown in this portion of the chart) signify outside processes.
This article is part of a series. For other articles in the series, click the following:
Analyzing products, demand, margins, and routings, Analyzing product mix and volumes: Useful but insufficient, PQ$ Analysis: Why revenue matters in product mix segmentation, Why a timeline analysis of order history matters
Most job shops, knowingly or unknowingly, operate multiple businesses under one roof. All these “businesses” use the same workforce, the same planning strategies, the same suppliers, the same facility layout, and the same equipment. In reality, job shop managers could split their product mix into two or more segments, and each segment ideally should be allocated a separate area and run using different management policies; support systems; workforce skills; and operational strategies, including scheduling, purchasing, inventory control, and facility layout. Why? It is because these segments share resources--including people and machines--and so inherently interfere and compete with each other.
As discussed in August, the PQ Analysis--relating product mix (P) to associated quantities (Q)--is a useful but incomplete method for segmenting the product mix of a job shop. Yes, it is simple to implement on a spreadsheet, and it complements value stream mapping (VSM). Although VSM and the PQ Analysis complement each other, both are really effective only in low-mix, high-volume situations. This method incorrectly focuses attention on a small percentage of a job shop’s product mix. Worse, it fails to consider the routing, revenue, stability, and repeatability of demand for a job shop’s different products. VSM works best in low-mix, high-volume assembly facilities. In fact, the fundamental concept of VSM is based on the theory of assembly line balancing--and an assembly line simply is not a job shop, and never will be. VSM can map a single or, at best, two or three routings, which is obviously not enough for a job shop. Nor have I seen VSM able to depict an entire family of products with different setups and cycle times for various operations in their routings.
Essentially, VSM is a method that misleads job shops into believing that, just because they have a few high-volume products, their entire business can run to the assembly line-like “beat” of repetitious production. This is why the PQR$T Analysis can play a valuable role--it reflects the realities job shop managers face. They have a diverse product mix (P) with different routings (R) produced in various quantities (Q) with varying revenue and profits ($) and demand stability and order repeatability (T). Analyzing all these factors helps shop managers see the whole picture.
Analyzing Products and RoutingsIn this column I describe an approach that incorporates a PR Analysis (one relating product mix and routing similarities) into a PQ Analysis, creating the PQR Analysis. I also show the benefits of a PQ$ Analysis, which brings revenue into the decision-making. Although not as comprehensive as the complete method of PQR$T Analysis, the product-quantity-routing-revenue (PQR$) analysis, will surely not mislead job shop owners about their product mix, as does the PQ Analysis.
The PQR Analysis segments a product mix into at least two, three, or more segments. Thereafter, product families in each segment can be produced using a suitable manufacturing system, be it a high-volume cell devoted to a single product; a flexible line devoted to a product family; or a flexible cell that, say, can run unattended overnight to produce a large family of parts, but during the day produce the remaining low-volume products in the product mix.
Much of the methodology behind PQR Analysis comes from the Production Flow Analysis (PFA) work of Prof. John Burbidge of Britain, as well as the Group Technology strategies that helped transform numerous job shops in the U.K. into cell-based facilities during the 1970s. These proven approaches for high-mix, low-volume manufacturing were pioneered by the British, Germans, and Russians--and not Toyota. The Toyota Production System provides significant value for high-volume, low-mix production. But a job shop, of course, is not Toyota.
The Analysis
Consider one job shop that has 530 products with routings using 57 pieces of equipment (work centers), including equipment available at the shop’s suppliers. Like any contract manufacturer, it’s a complex, highly variable operation.
The shop uses analytical software to compute the current state of affairs. In this example, the company uses the Production Flow Analysis and Simplification Toolkit (PFAST) from The Ohio State University, but it may well be possible to implement these same analytics using Microsoft Excel® or Access®, or even Six Sigma statistical analysis software such as Minitab®.
To start, the improvement team assigns each work center (W/C) a number and determines whether the machine is a monument or if it can be relocated (see Figure 1). They determine if the process would be expensive to duplicate if the shop wanted to purchase new machines to minimize shared resources among product-mix segments.
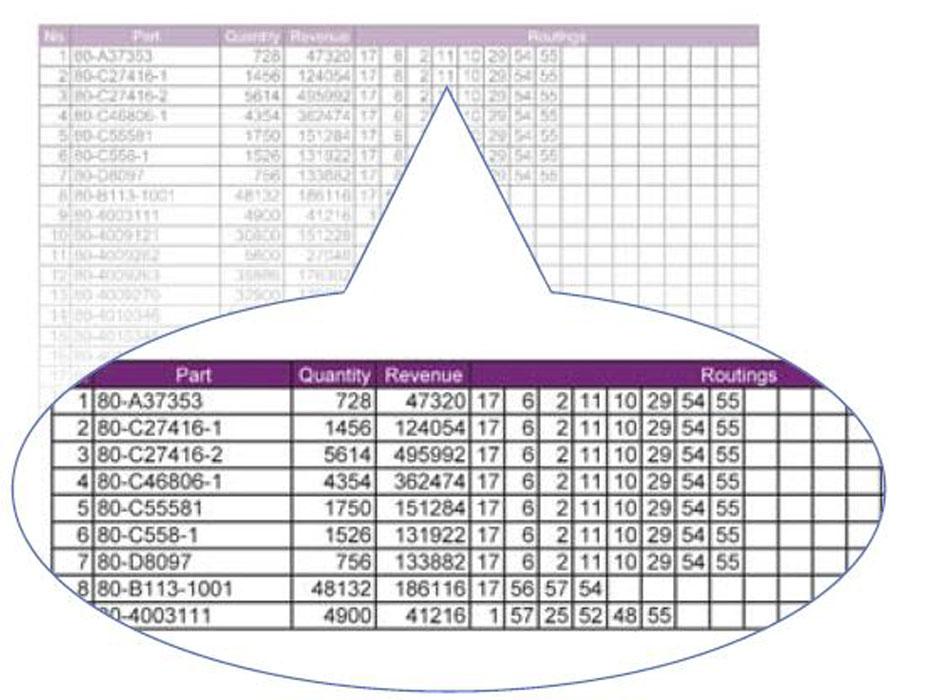
Figure 2
This spreadsheet shows the input data used for PQR$ Analysis; that is, quantity (Q), revenue ($), and the routing (R) for each product/part (P). Note, this is just a sample of parts that was extracted from the product mix of 530 part numbers, as described later. A complete PQR Analysis would include all 530 part numbers--and analyzing all product routings does have value, as revealed in the “Narrowing the Analysis” section.
The team also produces the information in Figure 2, which shows the quantity, routing (with corresponding W/C numbers), and revenue for each product number over a specified period. Figure 3 shows the current facility layout with all the W/C locations, including unmovable monuments.
Next the shop analyzes all 530 products and their individual routings to assess if there are families of parts using groups of machines that could be moved into manufacturing cells. From here they develop a full-scale PR Analysis (see Figure 4), also called a machine-part matrix clustering or product-process matrix clustering. Think of this analysis as a visualization of all 530 value streams without using VSM at all.
At every point a part number along the Y axis (a row in the spreadsheet) is matched with a work center along the X axis (a column in the spreadsheet), a “1” is placed. Compact blocks of 1s suggest part families are present. Note that Figure 4 is a 530-line chart miniaturized, so clusters of 1s appear as solid blue blocks.
Narrowing the Analysis
Working with all 530 part numbers at once isn’t practical for any improvement effort. So from here the team extracts a smaller sample of part numbers that have significant volume, significant revenue, or share common routings. Here is where analyzing the product mix (P), quantities (Q), routings (R), and revenue ($) comes into play. This is where the team also uses the PFAST software to replace the traditional PQ Analysis of the entire product mix with a PQ$ Analysis.
Figure 5 shows a typical PQ Analysis distribution of production volumes for a job shop. The head on the left side of the graph shows the few product numbers with high volumes, while the long tail to the right shows numerous jobs with low volumes.
Figure 6 brings revenue into the mix. As shown, most jobs have low quantities and low revenue--no surprises there. But note the remaining jobs. A few high-quantity jobs produce only moderate levels of revenue, while a significant number of low-quantity jobs produce moderate and even high revenue.
Considering both part quantity and revenue, the team focuses on products produced in quantities greater than 1,800 and earning revenue of more than $30,000, as shown by the dotted line in Figure 6. Initially only 44 product numbers were included in the sample, which accounts for only about 8 percent of the total product mix, 68 percent of the total volume, and 46 percent of the total revenue. In other words, almost half of the company’s earnings come from those 44 products.
Here is where that original, complete PQR Analysis in Figure 4 steps back into the picture. Of the hundreds of remaining products not included in the sample, 35 have routings similar or identical to a significant number of routings for the 44 products initially selected in the sample. Seeing this, the team decides to include them in the sample selected for improvement, bringing the total number of products to 79--or 15 percent of the total number of active products, about 74 percent of the total volume, and about 54 percent of the total revenue.
Analyzing the Sample
These steps narrow the analysis and determine which products use similar machines, but they do not truly reveal the complex material flow that plagues the job shop. So from here the team uses the product mix, quantity, and routing information for the 79 products in the sample to develop a “from-to” chart (see Figure 7).
The chart lists the same set of work centers in the same sequence on both the X and Y axes. Software breaks up each routing into several from-to segments--that is, the quantity of products that move from one specific W/C to another. If a part goes from W/C 17 to 6, 2, 11 and so on, the software will split this into “from-to” segments: 17 to 6, 6 to 2, 2 to 11, and so forth. Note that this analysis then has to be repeated for each of the 79 routings in the sample. (Not surprisingly, job shops shy away from doing such crucial analysis because of the tedium and inherent error of data mining by hand.)
For instance, Part No. 80-A37353 has a quantity of 728. The routing starts at W/C 17 and moves to W/C 6--that is, from the band saw to the furnace--before moving to other operations downstream. In this case, the quantity of 728 is inserted where W/C 17 (on the Y axis) and 6 (on the X axis) intersect, as shown in Figure 7. When this 728 is added to the quantities from all part numbers flowing between those machines, the chart reveals that, during a predetermined time period, 34,370 workpieces travel from the band saw (W/C 17) to the furnace (W/C 6).
When the flow volumes and directions between all pairs of work centers are superimposed on the facility layout, with arrow thicknesses proportional to traffic volumes, the result is the flow diagram in Figure 8. The chaos in this diagram is common for job shops.
Identifying Product Families
After analyzing the part routings using one of the four PR Analysis algorithms available in PFAST software, the team organizes the 79 part numbers into four product families, as shown in Figure 9. The clusters of 1s indicate products with similar routings using the same W/Cs.
In Figure 3 the blocks colored red correspond to the W/Cs that are monuments, and the blocks colored green correspond to outside operations (vendors and the company’s own machine shop located several miles away). Figure 9 uses the same color scheme--red for monuments and green for outside processes.
Using the results of Figure 9, the company develops a scatter plot depicted in Figure 10 to compare the four part families with each other, based on the total quantity (Y axis) and the total revenue (X axis). Then they develop another material flow diagram, as shown in Figure 11, that superimposes the material flow diagrams for each of the four part families on each other.
The Payoff
Seeing all this, could this shop be organized into four product family cells? Probably not, especially considering the number of monuments in part families 2 and 3 (again, those columns marked in red), although at least the first part family could be produced in a dedicated cell. Regardless, the analysis gives a clear picture of the current, albeit complex, state of affairs on the shop floor.
At first glance, all this may seem like a lot of effort for not much reward. But this analysis is just a starting point. It gives the improvement teams a guide, showing what to tackle first and which strategies would pay off, as well as which ideas to avoid.
The actual job shop in this example moved certain machines to reduce job travel time and purchased other equipment to make material flow more efficiently. Ultimately, the resulting throughput increase saved $137,000 a year. That’s not a bad return on investment.
Applying the Analysis
- Lay the foundational data for improvement. Kaizen event teams usually don’t have time to collect relevant data for all products. But with analyses already complete, the team could easily use just the PQ Analysis to segment their product mix into at least two areas, high volume and low volume. Then, using the PR Analysis for at least the high-volume segment, they could seek product families in that segment. Any product family found in that segment could then be the focus of a high-impact kaizen to implement a cell. A cell is ideal for fostering self-directed teamwork, for developing a cross-training plan, and developing leadership skills among the cell operators.
- Split the facility. With part families identified, the job shop could have one or more cells producing a family of products with similar routings. For one product family, these cells could entail an automated, flexible manufacturing cell capable of producing a family of parts in one or two setups. Another cell could focus on a product family of prototype and one-off jobs. Ideally, these high-margin jobs could be produced in a separate department.
- Absorb low-volume products into high-volume part families. The PQR$ Analysis can be applied to the low-volume segment. Low-volume, high-margin products can be grouped into those existing high-volume product families--if they share similar routings. As the manufacturing cell or improvement efforts directed at this product family make it even less costly to produce, the sales team can hunt for parts that also fit this product family. Some job shops even have purchased other product line manufacturers after they discover that its product or subcomponents already fit into their shop’s existing product families.
- Cull the product mix. For low-volume products that do not belong in any product family, a team can analyze their profitability. Conducting the PQR Analysis can determine if any product cannot indeed fit into other product families. If it cannot, the team can ask some questions: Does this low-volume product go to customers who provide high-volume work? Or is it complex fabrication or prototype work offering high margins? If so, that low-volume product may be worth keeping. If not, then it may be a product ripe for culling. As one plant manager at a sheet metal job shop once told me, “We gladly recommend to those customers that send us difficult and low-margin products that they go to our competitors with their business.”
- Implement “milk runs” for material replenishment. Scheduling deliveries of materials to manufacturing cells designed to fulfill orders for product families can be done using timed routes--that is, milk runs--performed by designated handlers. These people (called water spiders or water striders in lean parlance) could have an expanded role as “virtual cell managers”--that is, even though machines a strider monitors and supplies are dispersed, all those machines still produce a family of parts, and so their operators report to this roving “manager on wheels.”
- Competing through collaboration. Two competing job shops can work together. Say a shop identifies low-volume, less profitable products it wants to cull. Still, for another job shop, this product may fit into product families and be more profitable. In fact, that other job shop could be in the same situation. So in this case, these two companies could swap jobs, leaving both firms with more profitable work that better fits their operations. Of course, both shops must ensure that any swapping does not affect their market-diversity goals, and they must ensure that their new product acquisitions fit the capabilities of their existing (or planned) cells. Here, strategic planning done using PQR$ Analysis can play a central role
About the Author
subscribe now
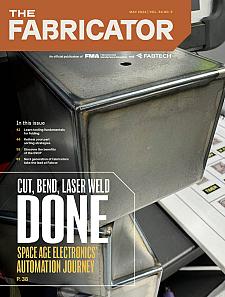
The Fabricator is North America's leading magazine for the metal forming and fabricating industry. The magazine delivers the news, technical articles, and case histories that enable fabricators to do their jobs more efficiently. The Fabricator has served the industry since 1970.
start your free subscription- Stay connected from anywhere
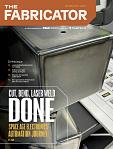
Easily access valuable industry resources now with full access to the digital edition of The Fabricator.
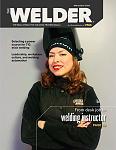
Easily access valuable industry resources now with full access to the digital edition of The Welder.
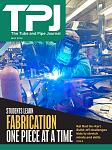
Easily access valuable industry resources now with full access to the digital edition of The Tube and Pipe Journal.
- Podcasting
- Podcast:
- The Fabricator Podcast
- Published:
- 04/16/2024
- Running Time:
- 63:29
In this episode of The Fabricator Podcast, Caleb Chamberlain, co-founder and CEO of OSH Cut, discusses his company’s...
- Trending Articles
Tips for creating sheet metal tubes with perforations
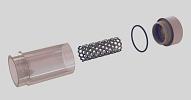
Are two heads better than one in fiber laser cutting?
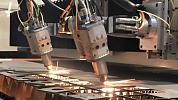
Supporting the metal fabricating industry through FMA
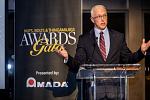
JM Steel triples capacity for solar energy projects at Pennsylvania facility
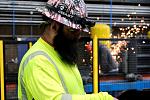
Omco Solar opens second Alabama manufacturing facility
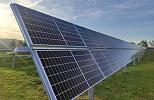
- Industry Events
16th Annual Safety Conference
- April 30 - May 1, 2024
- Elgin,
Pipe and Tube Conference
- May 21 - 22, 2024
- Omaha, NE
World-Class Roll Forming Workshop
- June 5 - 6, 2024
- Louisville, KY
Advanced Laser Application Workshop
- June 25 - 27, 2024
- Novi, MI