President
- FMA
- The Fabricator
- FABTECH
- Canadian Metalworking
Categories
- Additive Manufacturing
- Aluminum Welding
- Arc Welding
- Assembly and Joining
- Automation and Robotics
- Bending and Forming
- Consumables
- Cutting and Weld Prep
- Electric Vehicles
- En Español
- Finishing
- Hydroforming
- Laser Cutting
- Laser Welding
- Machining
- Manufacturing Software
- Materials Handling
- Metals/Materials
- Oxyfuel Cutting
- Plasma Cutting
- Power Tools
- Punching and Other Holemaking
- Roll Forming
- Safety
- Sawing
- Shearing
- Shop Management
- Testing and Measuring
- Tube and Pipe Fabrication
- Tube and Pipe Production
- Waterjet Cutting
Industry Directory
Webcasts
Podcasts
FAB 40
Advertise
Subscribe
Account Login
Search
What manufacturers should do with data after collecting it
Smart manufacturing requires accurate statistical analysis based on quality data, expert oversight
- By Bill Frahm
- November 17, 2023
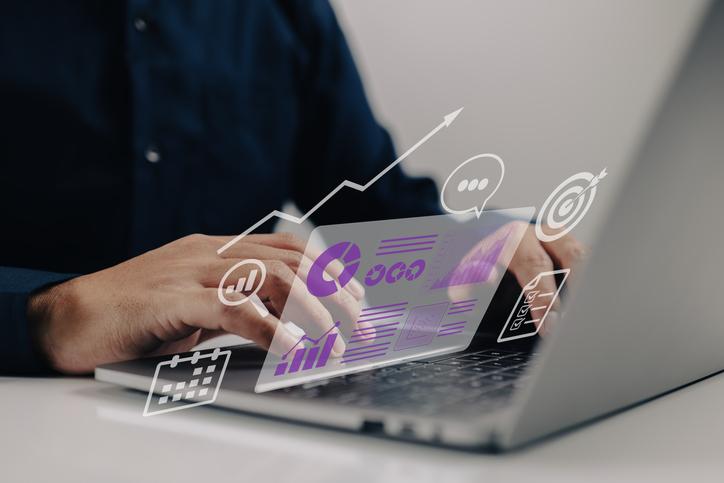
Collecting data does a company nothing. Knowing what to do with the data is the 'smart' part of smart manufacturing. ipuwadol/iStock/Getty Images Plus
Data is king is a popular mantra. Manufacturers collect information on equipment, inventory, products, and events; buy new devices to collect more of it; and sometimes boast about the quantity of data they hold. Your data is the foundation your smart manufacturing system builds upon.
Data can be structured, such as traditional application information in rows and columns on a spreadsheet, or unstructured, including photos, videos, and text documents. But the data itself is inert. It is a digital artifact representing a thing or an event. Beyond that representation, it does nothing but occupy space on a magnetic device.
Statistical Analysis
What brings value to that data is statistical analysis. The math categorizes, prioritizes, determines distributions, and discovers relationships through data selection, defining your representative population, categorizing information, analyzing information, and reporting findings and results.
Analysis helps you learn about your materials, equipment, and operations and find trends, relationships between variables, and causes and effects. In manufacturing, these insights help you specify materials and components, choose operational values, manage logistics, maintain reliability, and measure the efficiency of your operations.
Today’s AI systems can analyze both structured and unstructured data. These tools can identify relevant pieces of unstructured data based on keywords and geometry, allowing it to be included in analysis.
And the value of all this analysis depends on the integrity and relevance of your data, because data is king.
Subject Matter Expertise
But neither the data nor the math has real value unless guided and understood by subject matter experts (SME). These skilled employees understand the meaning of data sets and data elements and comprehend the nuances, capabilities, and weaknesses of data. They know the issues to be resolved and often have a general idea of where the problems may be originating. They are your only real source of reliable sample populations.
Your greatest asset when using smart manufacturing is a knowledgeable, confident, and engaged workforce. Without a skilled workforce, smart manufacturing risks becoming a pointless, random disaster. Uninformed employees, too frequently auditors, have created costly disasters through their misuse of analysis. Business history includes incidents in which SMEs weren’t included in the decision process and the results were disastrous. From Allied Crude Vegetable Oil Company to Enron, reckless and incompetent audit standards resulted in the perpetration of frauds large enough to rattle Wall Street.
You can build effective SME control by training and supporting your workforce. Your people should be able to communicate with data analysts, offer advice on data elements and their purpose, and review analytical results. SMEs must also be willing to challenge questionable results. Frequently, errors happen because nobody asked.
Data is biased by previous decisions: Equipment data reflects prior maintenance, setup, configuration, and operating decisions, and transactional data reflects your policies in operations, logistics, and scheduling. Your SMEs are critical to successful smart manufacturing, offering your best line of defense against both unintentional and intentional misapplication of data and statistical analysis.
Audit and Review
Audit and review of smart manufacturing must be dramatically different from traditional accountant-driven auditing. Old checklist- and paperwork-style audits become obsolete in the new systems environment. Algorithmic auditing is likely to advance, conducted by systems experts who understand the complexities of statistics, data integrity and bias, networks, and AI.
AI removes transparency from elements of your manufacturing operation and can modify algorithms to adapt to lessons learned. The problem is that these changes happen silently. Even AI developers claim that we really don’t fully understand what happens “under the hood.”
Therefore, frequent monitoring is required for algorithm development and system training, algorithm verification, changes resulting from machine learning, data integrity and sources of data bias, monitoring and calibration activities for monitoring and measurement devices, access controls, and standards for access to external data sources.
Training and Diligence = Discovery
What truly rules in smart manufacturing is maintaining a purpose of discovery. Combining hardware, advanced networks, and statistical capabilities, you can dig deeper into equipment history, events, and properties, learning quickly why you succeed and sometimes fail. Smart manufacturing can predict events, allowing for equipment maintenance that can better support reliable manufacturing, identify efficiencies in operations and logistics, and identify production failures.
In the complex world of smart manufacturing, training and diligence are mandatory to benefit from available opportunities. Your workforce must be prepared to work interactively with systems and data technologies. Employees must be able to understand analytical results and be able to respond appropriately. They must also feel empowered to question results that seem contrary to their own experience and knowledge.
subscribe now
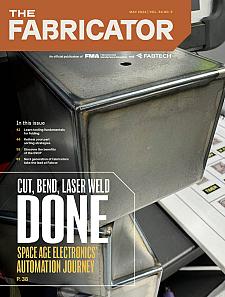
The Fabricator is North America's leading magazine for the metal forming and fabricating industry. The magazine delivers the news, technical articles, and case histories that enable fabricators to do their jobs more efficiently. The Fabricator has served the industry since 1970.
start your free subscriptionAbout the Author
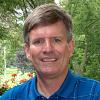
Bill Frahm
P.O. Box 71191
Rochester Hills, MI 48307
248-506-5873
- Stay connected from anywhere
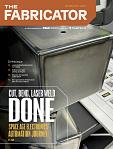
Easily access valuable industry resources now with full access to the digital edition of The Fabricator.
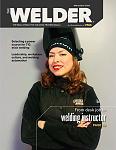
Easily access valuable industry resources now with full access to the digital edition of The Welder.
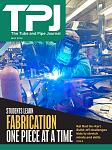
Easily access valuable industry resources now with full access to the digital edition of The Tube and Pipe Journal.
- Podcasting
- Podcast:
- The Fabricator Podcast
- Published:
- 04/16/2024
- Running Time:
- 63:29
In this episode of The Fabricator Podcast, Caleb Chamberlain, co-founder and CEO of OSH Cut, discusses his company’s...
- Trending Articles
Tips for creating sheet metal tubes with perforations
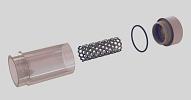
Supporting the metal fabricating industry through FMA
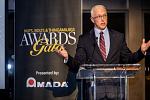
JM Steel triples capacity for solar energy projects at Pennsylvania facility
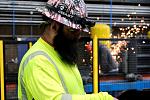
Are two heads better than one in fiber laser cutting?
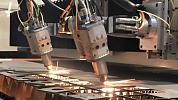
Fabricating favorite childhood memories
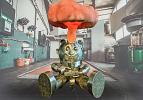
- Industry Events
16th Annual Safety Conference
- April 30 - May 1, 2024
- Elgin,
Pipe and Tube Conference
- May 21 - 22, 2024
- Omaha, NE
World-Class Roll Forming Workshop
- June 5 - 6, 2024
- Louisville, KY
Advanced Laser Application Workshop
- June 25 - 27, 2024
- Novi, MI