President
- FMA
- The Fabricator
- FABTECH
- Canadian Metalworking
Categories
- Additive Manufacturing
- Aluminum Welding
- Arc Welding
- Assembly and Joining
- Automation and Robotics
- Bending and Forming
- Consumables
- Cutting and Weld Prep
- Electric Vehicles
- En Español
- Finishing
- Hydroforming
- Laser Cutting
- Laser Welding
- Machining
- Manufacturing Software
- Materials Handling
- Metals/Materials
- Oxyfuel Cutting
- Plasma Cutting
- Power Tools
- Punching and Other Holemaking
- Roll Forming
- Safety
- Sawing
- Shearing
- Shop Management
- Testing and Measuring
- Tube and Pipe Fabrication
- Tube and Pipe Production
- Waterjet Cutting
Industry Directory
Webcasts
Podcasts
FAB 40
Advertise
Subscribe
Account Login
Search
Harnessing the power of machine learning in metal forming
How manufacturers can use data to improve operations, and why AI differs from machine learning
- By Bill Frahm
- May 10, 2019
- Article
- Automation and Robotics
One of the more interesting, misunderstood, and misrepresented components of the industrial internet of things (IIoT) is artificial intelligence (AI), a term that came into use in the middle 1950s. Early AI was used for advanced problem- solving and symbolic methods. Today’s AI takes advantage of powerful processing capability, along with our ability to store large amounts of data on affordable equipment.
Increasingly, the term AI is being overused by marketers to the point of being meaningless. In the context of manufacturing, most IIoT applications actually use machine learning. Machine learning identifies the ability of software to change its behavior based on observations taken from data.
Given large amounts of data, machine learning software can analyze and discover context and patterns within your manufacturing operations. A subset of machine learning, deep learning, employs artificial neural networks to discover patterns in events and behaviors. Once a pattern is discovered, it is assigned a probability. If the probability is acceptable, the equipment will adapt its behavior to the new input.
Contrary to reports by popular media (and “Terminator” movies), machine learning capabilities cannot remotely compare to the ability of humans to observe and learn. The human brain has billions of nodes. Artificial neural networks come nowhere close in both the number of nodes and their observational capabilities.
Machine learning offers manufacturers opportunities to use data to improve operations, assist with predictive and condition-based maintenance, and manage logistics:
- Machine learning allows your plant to monitor and improve equipment energy use and emission efficiency in such systems as forming machines, ovens, and power generation. Continuous, deep learning can allow your plant to both reduce energy costs and reduce your environmental impact.
- Predictive maintenance requires a lot of observation and math to develop a machine life cycle curve, predict failures, and schedule maintenance tasks. Machine learning allows you to determine accurate equipment life cycle curves based on many observations of actual experience. Machine learning and condition monitoring can alert you to conditions that will lead to failure. As you learn, you can predict the length of time between an unfavorable condition and quality, production, or safety issues and schedule maintenance tasks appropriately.
- The semiconductor industry has used machine learning effectively to identify the root causes of quality failures and increase production yield. The result is less scrap, less testing, and greater productivity.
The challenge presented by technology that learns and adapts, but has only limited vision, imposes five main requirements on implementation managers:
- Develop and maintain proficiency in your manufacturing operations – To incorporate flexible technology on your plant floor, you need to understand the fundamental properties of the materials you use, the behaviors and risks of your forming technologies, and the capabilities and limitations of your manufacturing processes. Only with complete understanding of your forming environment can you reliably define the capabilities and limitations of your new technologies.
- Define rules for use and engagement of AI during human intervention – Well-trained employees with superior observation skills have better judgment than software. When a conflict exists between your software and operators, you must define who will get priority in each situation and how conflicts should be reviewed and resolved.
- Prioritize human safety – No system should be permitted to place human life at risk. A system given any amount of autonomy introduces the risk of unanticipated consequences. Avoiding human risks requires such actions as training employees in safe operation and maintenance around partially autonomous equipment, preventing AI from operating compressive or rotating equipment while safeties are set, and preventing release of VOCs and toxic fluids.
- Understand data persistence and analysis – Among the decisions you will have to make are what events to store from each monitor. Your monitors will record events and state changes during operation. You may also want to record operations events that affect the equipment and components you are monitoring.
- Ensure the integrity of your data over its life cycle -- Machine learning must be accurate to offer value and prevent safety and performance issues. Wireless communication is a valuable and affordable tool in advanced manufacturing systems. Implementing wireless in a manufacturing environment can be challenging, though. Before installing a wireless network, you must measure your study, measure your radio environment, and identify alternatives to ensure data integrity.
Machine learning is a promising technology that offers tremendous opportunities for manufacturers, and it’s still emerging from the laboratory. Capabilities are expanding rapidly, but challenges still exist. While machine learning can help you improve your operations, you must approach it cautiously. The risks to production and safety can be managed, but only if you approach technology with your eyes open and remain aware of the risks.
About the Author
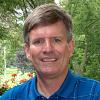
Bill Frahm
P.O. Box 71191
Rochester Hills, MI 48307
248-506-5873
subscribe now
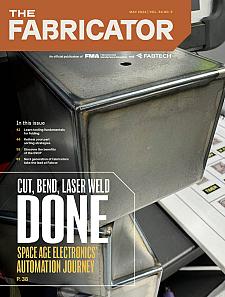
The Fabricator is North America's leading magazine for the metal forming and fabricating industry. The magazine delivers the news, technical articles, and case histories that enable fabricators to do their jobs more efficiently. The Fabricator has served the industry since 1970.
start your free subscription- Stay connected from anywhere
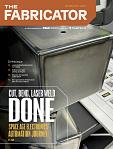
Easily access valuable industry resources now with full access to the digital edition of The Fabricator.
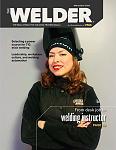
Easily access valuable industry resources now with full access to the digital edition of The Welder.
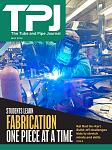
Easily access valuable industry resources now with full access to the digital edition of The Tube and Pipe Journal.
- Podcasting
- Podcast:
- The Fabricator Podcast
- Published:
- 04/16/2024
- Running Time:
- 63:29
In this episode of The Fabricator Podcast, Caleb Chamberlain, co-founder and CEO of OSH Cut, discusses his company’s...
- Trending Articles
Tips for creating sheet metal tubes with perforations
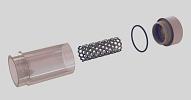
JM Steel triples capacity for solar energy projects at Pennsylvania facility
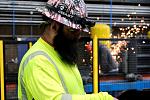
Are two heads better than one in fiber laser cutting?
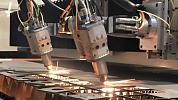
Supporting the metal fabricating industry through FMA
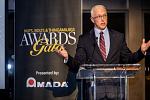
Omco Solar opens second Alabama manufacturing facility
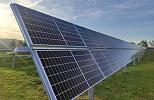
- Industry Events
16th Annual Safety Conference
- April 30 - May 1, 2024
- Elgin,
Pipe and Tube Conference
- May 21 - 22, 2024
- Omaha, NE
World-Class Roll Forming Workshop
- June 5 - 6, 2024
- Louisville, KY
Advanced Laser Application Workshop
- June 25 - 27, 2024
- Novi, MI